The role of AI in fraud detection in financial services
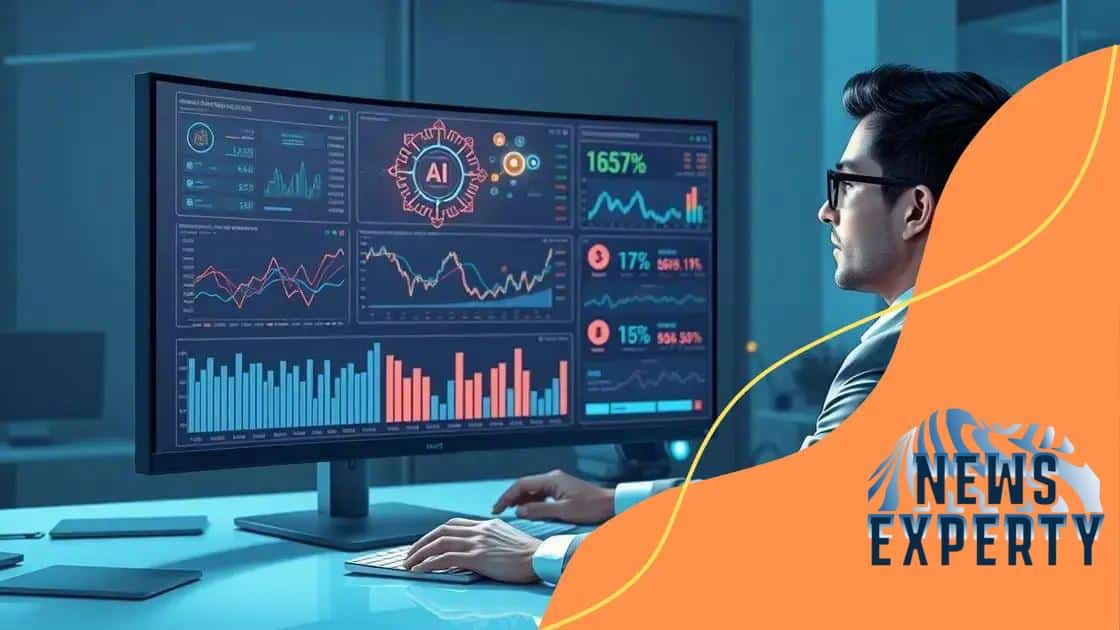
The role of AI in fraud detection in financial services includes real-time monitoring, automated alerts, and advanced analytics to quickly identify and mitigate fraudulent activities, enhancing security and customer trust.
The role of AI in fraud detection in financial services is transforming how institutions combat deceitful activities. Have you ever wondered how banks stay ahead of fraudsters? Let’s dive deep into this fascinating integration of technology.
Understanding financial fraud dynamics
Understanding the dynamics of financial fraud is essential for creating effective detection strategies. Fraud in financial services can take many forms, from identity theft to fraudulent transactions. It’s crucial to recognize these variations to combat them effectively.
One key aspect of financial fraud is the evolution of techniques employed by fraudsters. As technology advances, so does their ability to exploit vulnerabilities. Financial institutions must stay vigilant and adapt their defenses accordingly.
The Types of Financial Fraud
Several common types of fraud exist, and understanding their characteristics can aid in prevention:
- Identity Theft: This occurs when someone unlawfully obtains and utilizes another person’s personal information to commit fraud.
- Credit Card Fraud: This happens when a fraudster uses someone else’s credit card information to make unauthorized purchases.
- Loan Fraud: Individuals may provide false information to secure loans they don’t intend to repay.
- Wire Transfer Fraud: Scammers trick individuals or businesses into transferring money under false pretenses.
Awareness of these fraud types helps create a framework for detection. For instance, institutions can implement advanced monitoring systems that track unusual activities closely.
Furthermore, the nature of fraud is constantly changing. Technology plays a significant role in this evolution. As criminals innovate, so must the tactics used by financial institutions. By employing artificial intelligence, these institutions can analyze vast amounts of data quickly and accurately. Such analysis can reveal hidden patterns that may indicate fraudulent activity, enabling prompt responses.
Behavioral Indicators of Fraud
Tracking behavioral changes can also provide clues. For example, if a customer normally conducts minimal transactions but suddenly initiates large withdrawals, it could trigger alerts for potential fraud. Similarly, abnormal purchasing habits can serve as a warning sign. Recognizing these behaviors is crucial in maintaining security.
Education and awareness among employees and customers further serve to deter fraud. Training staff to identify suspicious activities enhances both internal security measures and customer service. Additionally, customers should be informed about how to protect themselves from potential fraud attempts.
With the increasing sophistication of fraud, adapting to new trends is only part of the solution. Financial institutions must prioritize ongoing education and the refinement of their technological tools to remain a step ahead of fraudsters.
How AI analyzes transaction patterns
AI’s ability to analyze transaction patterns is revolutionizing the way financial institutions detect fraud. By leveraging advanced algorithms, AI systems can sift through large datasets quickly, identifying unusual behavior that may indicate fraudulent activity.
This technology enables a broader understanding of transaction flows. Financial institutions can access vast amounts of data generated daily. With AI, they can monitor these transactions in real time, making it possible to spot anomalies as they occur. This is crucial because fraud is most often successful when it goes unnoticed for extended periods.
Key Techniques Used in AI Analysis
Several techniques enhance the effectiveness of AI in analyzing transaction patterns:
- Machine Learning Algorithms: These algorithms learn from historical transaction data. They build models that help predict potential fraud based on past patterns.
- Anomaly Detection: AI identifies deviant behaviors within transactions, flagging occurrences that deviate from established customer profiles.
- Clustering Techniques: By grouping similar transaction data, AI can highlight unusual clusters that require further investigation.
- Behavioral Analytics: This involves understanding user behavior through their transaction history, helping to predict future actions.
As AI continues to learn, its detection capabilities improve. It analyzes vast amounts of transaction data, converting this information into actionable insights. For example, if a customer typically conducts transactions during business hours and suddenly makes a purchase late at night in a different country, AI can flag that transaction for review immediately.
Furthermore, the integration of AI tools can significantly reduce the time needed to investigate potential fraud. Instead of spending hours sifting through data, analysts can focus on high-risk transactions highlighted by the AI. This efficiency allows for quicker responses to potential threats, enhancing overall security.
The collaboration between AI and human analysts can create a robust defense against fraud. While AI adeptly analyzes patterns and suggests areas of concern, human intuition and expertise play a critical role in validating these findings. Together, they form a comprehensive approach to fraud detection.
Real-time alerts and automated responses
Real-time alerts and automated responses are essential components in the fight against financial fraud. These tools help institutions respond to suspicious activity swiftly, minimizing potential losses and protecting customers.
With the rise of digital transactions, the speed at which alerts are generated has become crucial. Financial institutions can now monitor transactions in real-time, allowing them to detect irregularities as they arise. By incorporating advanced algorithms, AI systems identify patterns that may suggest fraudulent behavior, sending alerts to the relevant personnel or systems.
How Real-Time Alerts Work
The process begins when a transaction occurs. As data is analyzed, the system looks for anomalies. If something seems off, an alert is triggered immediately. For example, if a customer usually makes small purchases but suddenly attempts a big transaction, the system can flag this for review.
Some key benefits of real-time alerts include:
- Immediate Action: Institutions can act quickly to prevent fraud before a significant loss occurs.
- Customer Protection: Clients receive notifications about unusual activity, allowing them to verify transactions.
- Reduced Risk: By catching fraud early, institutions minimize their overall exposure.
- Increased Trust: Customers feel more secure knowing that their financial institutions are actively monitoring their accounts.
Automated responses further enhance the effectiveness of these alerts. For instance, if a transaction is flagged, the system can temporarily freeze the account or request additional verification from the customer. This response can prevent unauthorized actions while the situation is assessed.
Moreover, automated systems can learn over time. As they gather data from alerts and responses, they become increasingly adept at identifying genuine threats, leading to fewer false alarms. This evolution improves efficiency and allows human analysts to focus on more complex issues.
Combining real-time alerts with automated responses creates a proactive approach to fraud detection. It allows institutions to protect their assets and maintain customer trust in an ever-evolving digital landscape.
Case studies of AI in action
Case studies of AI in action provide valuable insights into how financial institutions effectively combat fraud. By examining real-world applications, we can understand the significant impact AI technology has on enhancing fraud detection efforts.
One notable example comes from a leading bank that implemented an AI-powered fraud detection system. This bank utilized machine learning algorithms to analyze transaction data across millions of accounts. By establishing a baseline of normal customer behavior, the AI could identify transactions that deviated from the norm. For instance, if a regular customer suddenly made a large purchase in a foreign country, the system flagged it for further investigation.
Success Stories of AI Adoption
Several financial institutions have shared success stories illustrating how AI has transformed their fraud detection processes:
- Bank of America: By using AI, they reduced fraud-related losses by over 30%. Their system analyzes transaction patterns in real time, enabling quick responses to suspicious activities.
- HSBC: This global bank integrated AI tools to detect money laundering activities. Their solution has identified numerous suspicious transactions, significantly minimizing financial risks.
- American Express: With the deployment of AI, they enhanced their ability to identify fraud while reducing false positives. Their system allows genuine transactions to go through without unnecessary delays.
- PayPal: Using AI-driven models, they can assess fraud risk in real time. Their system evaluates user behavior, ensuring that potentially fraudulent transactions are scrutinized promptly.
These case studies demonstrate the versatility and efficiency of AI in various financial sectors. Utilizing large datasets, AI systems learn and adapt, becoming more effective over time. As these institutions developed their AI capabilities, they also improved customer trust and satisfaction by providing smoother transaction experiences.
In addition to immediate fraud detection, AI has enabled long-term benefits for these organizations. They not only save money but also gain valuable customer insights that can inform future strategies. By analyzing patterns and trends, financial institutions can proactively address potential vulnerabilities.
Overall, the successful case studies highlight the crucial role AI plays in modern fraud detection. They serve as roadmaps for other institutions aiming to enhance their own security measures while building confidence in their services.
Future prospects of AI in fraud prevention
The future prospects of AI in fraud prevention are bright and filled with potential. As technology continues to advance, financial institutions are expected to adopt even more sophisticated tools to stay ahead of fraudsters.
One of the exciting developments is the creation of more adaptive AI systems. These systems can learn and evolve based on new data without needing constant human intervention. As they process large amounts of transaction data, they will refine their algorithms continually to enhance detection capabilities.
Emerging Trends in AI for Fraud Prevention
Several trends indicate where AI technology is headed in the realm of fraud prevention:
- Predictive Analytics: AI will increasingly utilize predictive analytics to foresee potential fraud patterns before they emerge. This allows institutions to take preemptive action against suspected fraud.
- Natural Language Processing (NLP): NLP can help analyze customer communications. By understanding the context, AI can better identify deception or fraudulent intent in customer interactions.
- Blockchain Integration: Combining AI with blockchain technology may enhance transparency and traceability in transactions. This could create a more secure environment where fraudulent actions are easily traceable.
- Enhanced User Authentication: Future AI developments may include more advanced biometric verification methods, such as facial recognition or voice recognition, making unauthorized access harder for fraudsters.
As AI technology matures, it will also focus on minimizing false positives. This goal is crucial, as reducing unnecessary account freezes or customer inquiries leads to better customer experiences. Machine learning models will play a significant role in distinguishing between legitimate and suspicious activities with greater accuracy.
Moreover, the collaboration between humans and AI will improve. While AI can analyze data at incredible speeds, the intuition and creativity of human analysts are irreplaceable. Financial institutions will likely see more hybrid systems that leverage the strengths of both AI and human insight.
In summary, the future of AI in fraud prevention promises innovative solutions that adapt to changing tactics employed by criminals. Institutions that embrace these advancements will not only enhance their fraud detection capabilities but also build trust with customers who seek secure financial services.
FAQ – Frequently Asked Questions about AI in Fraud Detection
How does AI improve fraud detection?
AI enhances fraud detection by analyzing large volumes of transaction data quickly, identifying patterns, and flagging unusual behaviors in real time.
What are real-time alerts in fraud prevention?
Real-time alerts notify financial institutions immediately when suspicious transactions occur, allowing them to act quickly to prevent fraud.
Can AI reduce false positives in fraud detection?
Yes, AI systems continuously learn and adapt, improving their ability to distinguish between legitimate transactions and potential fraud, which reduces false positive alerts.
What is the future of AI in financial services?
The future of AI in financial services includes more sophisticated predictive analytics, improved user authentication methods, and better integration with technologies like blockchain.