The growing importance of data science in education systems
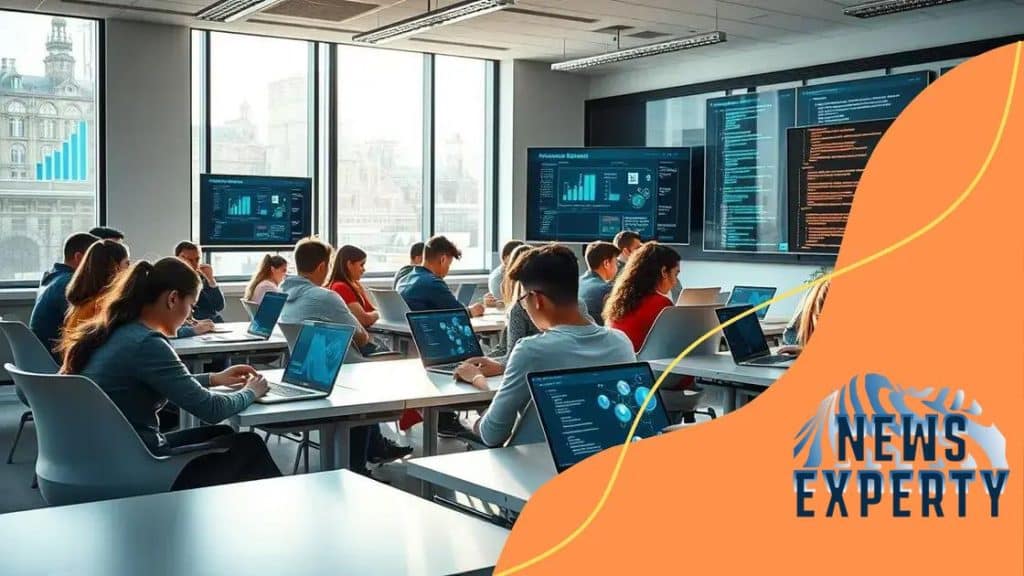
Anúncios
The growing importance of data science in education systems enhances personalized learning, improves student outcomes, and empowers educators to make informed decisions through data-driven strategies and AI integration.
The growing importance of data science in education systems is something we should all pay attention to. It’s fascinating how data shapes educational practices, isn’t it? In this article, we’ll dive into its impact on learning and analysis.
Anúncios
Understanding data science in education
Understanding data science in education is essential for transforming the way we teach and learn. It brings a wealth of tools and techniques that can help educators improve student outcomes. By leveraging data, schools can make informed decisions that lead to enhanced learning experiences.
Key Components of Data Science in Education
Data science involves several key components that are vital in the educational sector. Understanding these components helps in utilizing data effectively:
- Data Collection: Gathering information on student performance and engagement.
- Data Analysis: Interpreting data to find trends and insights.
- Data Visualization: Presenting data in a visual format for easier understanding.
- Predictive Modeling: Using data to forecast student success and areas for improvement.
As schools embrace data-driven decisions, they can identify challenges and successes more clearly. When educators analyze data, they can tailor their approaches to suit each student’s needs. This personalized learning experience is crucial in today’s diverse classroom environments.
Anúncios
The Role of Technology
Technology plays a significant role in facilitating data science in education. Various tools and software can analyze vast amounts of data quickly. For instance, learning management systems (LMS) track student progress and engagement. Educators can use this information to adapt their teaching methods and provide support where needed.
Moreover, the integration of artificial intelligence can enhance data analysis further. AI can help educators predict outcomes and recommend personalized learning paths that cater to individual students. By embracing such technologies, educational institutions can enhance their instructional strategies and create a more engaging learning environment.
Incorporating data science into education is more than just adopting new tools; it’s about creating a culture where data informs every decision. This approach encourages continuous improvement and fosters an environment where students can thrive. Educators who understand data science can lead this charge, ensuring that they and their students are well-equipped for the future.
Real-world applications of data science in schools
Real-world applications of data science in schools demonstrate how data can enhance educational practices. Schools are increasingly leveraging data to improve teaching methods and support student learning. By using data effectively, educators can make informed decisions that positively impact student performance.
Examples of Data Usage
Many schools are applying data science in various ways, leading to improved outcomes. Here are several examples:
- Personalized Learning: Educators use data to tailor learning experiences based on each student’s strengths and weaknesses.
- Curriculum Development: Data informs curriculum adjustments, ensuring it meets the needs of current students.
- Monitoring Attendance: Tracking attendance data helps identify patterns and implement strategies to improve student engagement.
- Assessment Analysis: Analyzing assessment data supports teachers in identifying areas where students struggle.
Data science also allows educators to predict student outcomes. For instance, by examining past data, schools can forecast which students may need extra support. This proactive approach helps to address issues before they become significant problems.
Enhancing Teacher Collaboration
Data science fosters better collaboration among teachers. With access to shared data, educators can discuss student progress and share successful strategies. This teamwork leads to a more cohesive educational experience for the students.
In addition, schools use data science to evaluate and improve their programs. By analyzing data on program effectiveness, they can make necessary adjustments to enhance learning. This ensures that resources are used wisely and effectively.
Overall, real-world applications of data science in schools are making a substantial difference. They not only support teachers but also improve student learning outcomes. The future of education will continue to evolve as more schools recognize the value of data-driven decision-making.
How data-driven decisions enhance learning
How data-driven decisions enhance learning is an important topic for educators. By utilizing data, teachers can better understand their students’ needs and adapt their methods accordingly. This approach not only benefits students but also empowers educators to make informed changes that improve the overall learning environment.
Understanding Student Needs
Data allows educators to pinpoint areas where students may struggle. For example, analyzing test scores, homework completion rates, and classroom participation helps identify patterns. These insights enable teachers to intervene early, providing targeted help to those who need it most.
- Identifying Learning Gaps: Teachers can discover specific topics where students may need more support.
- Tailoring Instruction: By understanding strengths and weaknesses, teachers can customize their lessons.
- Improving Student Engagement: Data can reveal which teaching methods resonate best with students, making lessons more engaging.
- Tracking Progress: Continuous assessment data helps track how students improve over time.
Moreover, with the insights gained, schools can implement support programs. For instance, if data shows that many students struggle with a particular subject, schools might introduce tutoring sessions or after-school workshops.
Creating Collaborative Learning Environments
Data-driven decisions also promote collaboration among educators. Sharing data allows teachers to work together, aligning their strategies to support students more effectively. When everyone is informed about student performance, the entire team can focus on helping individual students thrive.
Furthermore, data can guide professional development for teachers. Schools can analyze which teaching practices yield the best results and offer tailored training opportunities. By equipping teachers with the right tools and techniques, schools can enhance the quality of education provided.
In essence, data-driven decisions are transforming the educational landscape. By using data thoughtfully, educators can create customized, effective learning experiences that meet the diverse needs of their students.
Challenges of integrating data science in education
Integrating data science in education comes with various challenges that schools must address. While the benefits are clear, the hurdles can hinder progress and implementation. Understanding these challenges is crucial for educators aiming to enhance their teaching through data.
Data Privacy Issues
One major challenge is ensuring data privacy for students. Schools collect a vast amount of information, and protecting this data is paramount. Educators must comply with regulations that safeguard student information from breaches. Failure to do so can lead to serious consequences.
- Compliance Regulations: Schools must navigate laws like FERPA to ensure student data is handled appropriately.
- Parental Consent: Obtaining consent from parents before collecting student data can be a complex process.
- Data Security: Schools need robust security measures to prevent unauthorized access to sensitive information.
Moreover, balancing transparency with privacy can be challenging. Teachers need access to data to improve learning, but they must also respect student confidentiality.
Lack of Training for Educators
Another significant challenge is the lack of training for educators in data science. Many teachers are not familiar with how to analyze and interpret data effectively. Without proper training, the integration of data science can fall flat.
This gap in knowledge may lead to misinterpretation of data and, consequently, poor decision-making. Providing professional development opportunities is essential to equip educators with the skills they need to leverage data successfully.
Resistance to Change
Resistance to change is a common barrier in many schools. Teachers may be set in their ways and reluctant to adopt new technologies or methods. This resistance can stem from a variety of factors, including fear of the unknown and lack of confidence in using new tools.
To address this, school leaders should foster an open culture that encourages experimenting with data-driven approaches. Building a supportive environment where educators feel comfortable learning can help mitigate this challenge.
Finally, achieving alignment among various stakeholders—teachers, administrators, and parents—can be difficult. Each group may have different perspectives on how data science should be implemented in schools, leading to conflicts and confusion. Clear communication and collaborative planning are vital to overcoming these challenges.
Future trends in educational data science
Future trends in educational data science point towards a more integrated and personalized learning experience. As technology evolves, data science will become an even more powerful tool for educators and students alike. Understanding these trends can help schools prepare for the changes ahead.
Increased Use of Artificial Intelligence
One of the most significant trends is the increasing use of artificial intelligence (AI) in education. AI can analyze large sets of data quickly, providing insights that may not be immediately visible to educators. This capability will allow for more personalized learning experiences and help identify at-risk students early.
- Adaptive Learning: AI systems can adapt materials to fit each student’s learning pace, making education more effective.
- Automated Feedback: Students can receive instant feedback on their work, helping them improve more rapidly.
- Predictive Analytics: AI can forecast future student performance, enabling early interventions.
As more tools integrate AI, teachers will find it easier to utilize data effectively. This shift can lead to enhanced engagement and improved academic results.
Emphasis on Data Literacy
Another trend is the growing emphasis on data literacy among students and educators. As schools adopt data-driven strategies, understanding how to interpret and use data becomes essential. Future curricula will likely include a stronger focus on data literacy skills.
Schools may incorporate lessons and workshops that teach students how to analyze data responsibly. This knowledge not only helps in their education but also prepares them for future careers in a data-driven world.
Collaborative Platforms for Data Sharing
Collaboration will also play a crucial role in the future of educational data science. Schools are likely to adopt platforms that allow for sharing data among educators, administrators, and even parents. This transparency enhances communication and promotes collective efforts to improve student outcomes.
Such platforms can facilitate discussions about student progress and enable educators to share successful strategies. By working together, all stakeholders can better support students in their learning journeys.
In summary, future trends in educational data science will bring more personalized learning, heightened data literacy, and enhanced collaboration. By embracing these trends, schools can ensure they are effectively preparing students for the demands of tomorrow’s world.
FAQ – Frequently Asked Questions about Data Science in Education
How can data science improve student outcomes?
Data science can identify learning gaps, tailor teaching methods, and provide personalized learning experiences to enhance student performance.
What are the main challenges of integrating data science in schools?
Challenges include data privacy issues, lack of training for educators, and resistance to change among staff.
What role does artificial intelligence play in education?
AI helps analyze student data quickly, enabling adaptive learning, real-time feedback, and predictive analytics for early intervention.
How can schools foster data literacy?
Schools can implement curricula that teach students how to interpret and utilize data effectively, preparing them for future data-driven environments.